Article
Cover
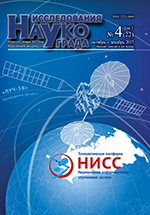
Title
Tools for visualization of time series in space researchAuthor
V.S. MaraevOrganization
Siberian Federal UniversityKrasnoyarsk, Russian Federation
Abstract
Time series analysis is a key step in building a prediction model. That is why it is very important to consider the data from the various parties, because the analysis helps to identify the various features and options under consideration of the time series. This is especially important when analyzing the data of space research, as often, their analysis does not lead to a fairly clear pattern. Therefore, data visualization is a powerful tool in this stage of the prediction model. Very often, for the visualization of time series in space research, only linear graphs are used that can’t represent the entire specifics of the series, so it is worthwhile to consider other methods and tools for visualizing space research data. This article analyzes the materials for time series visualization tools. The main visualization tools are considered, such as histograms, distribution density charts, box-and-whisker plots, heat maps, scatter and autocorrelation plots. These tools are demonstrated on examples of data obtained from space research. The advantages and disadvantages of various time series visualization tools in space research are revealed. The recommendations on the expediency of using these visualization tools in various situations are outlined. The corresponding conclusions are drawn on the basis of the conducted studies on the analysis of time series visualization tools in space research.Keywords
visualization, time series, space research, histogram, box and whisker plots, heat map, autocorrelation plotsReferences
[1] George E. P. Box, Gwilym M. Jenkins, Gregory C. Reinsel. Time Series Analysis: Forecasting and Control. Wiley Series in Probability and Statistics, 2013, pp. 92–161. ISBN 1118619064, 9781118619063.
[2] Kendall M., Stewart A. Multivariate statistical analysis and time series. Moscow, Nauka Publ., 1976, 736 p.
[3] Mishulina O. A. Statistical analysis and time series processing. Moscow, MIFI Publ., 2004, р. 38.
[4] Evdokimov I. V., Mikhalev A. S., Novikov O. S., Sukhanov A. V. Application of free licenses for software development in Russia. International Journal of Applied and Fundamental Research, 2017, no. 6-1, pp. 33–36.
[5] Evdokimov I. V., Yashchenkov K. G., Telkov A. Yu., Tataurov V. A. Expert methods for assessing the complexity of developing software projects. Economics and management systems management, 2017, vol. 24, № 2.2, pp. 272–276.
[6] Evdokimov I. V., Domantsevich V.S., Konyhov V.A. THE USE OF ONLINE APPLICATIONS FOR PROJECT MANAGEMENT FOR PLANNING IN IT-MANAGEMENT. Modern Information Technologies, 2017, no. 25 (25), pp. 44–47.
[7] International Center for Small Planets. Data Available from the Minor Planet Center. Available at: http://www.minorplanetcenter.net/ (accessed 30.10.2017).
[8] Center for Near-Earth Objects Dynamics. The AstDyS database [Electronic resource]. Available at: http://hamilton.dm.unipi.it/astdys/index.php?pc=2.0&o=094 (accessed 30.10.2017).
[9] International Meteor Organization. Visual Meteor Database [Electronic resource]. Available at: https://www.imo.net (reference date: 30.10.2017).
[10] Chronology of manned spaceflight. LAUNCHLOG [Electronic resource]. Available at: http://planet4589.org/space/log/launch.html (accessed 30.10.2017).
[11] The official open data storage of the Hubble Space Telescope. Hubble Space Telescope database [Electronic resource]. Available at: http://www.nasa.gov/hubble/ (accessed 30.10.2017).
[12] Ayvazyan S. A. Applied statistics. Fundamentals of Econometrics. Vol. 2. Moscow, Unity-Dana Publ., 2001, p. 432. ISBN 5-238-00305-6.
[13] Magnus Ya. R., Katyshev P. K., Peresetsky A. A. Econometrics. The initial course. Moscow, Case Publ., 2007, p. 504. ISBN 978-5-7749-0473-0.
[14] Econometrics. Ed. Eliseeva I. I. 2 nd ed. Moscow, Finances and Statistics Publ., 2006, p. 576. ISBN 5-279-02786-3.
[15] Woodward W. A., Gray H. L. & Elliott A. C. Applied Time Series Analysis. CRC Press, 2012, рp. 63–64.
[16] Hyndman Rob J., Athanasopoulos G. Forecasting: principles and practice. University of Western Australia, 2013, pp. 14–32. ISBN-13: 978-0987507105.
[17] Ayvazyan S. A., Buchstaber V. M., Enyukov I. S., Meshalkin L. D. Applied Statistics: Classification and Dimension Reduction. Moscow, Finance and Statistics Publ., 1989, 607 p.
[18] Shasha D. High Performance Discovery in Time Series. Springer, 2004, pp. 3–7.
[19] Cowpertwait P. S. P., Metcalfe A. V. Introductory Time Series with R. Springer, 2009, pp. 27–43.
[20] Eliseeva I. I., Yuzbashev M. M. General Theory of Statistics. Ed. I. I. Eliseeva. 4th edition, revised and enlarged. Moscow, Finance and Statistics Publ., 2002, 480 p. ISBN 5-279-01956-9.
For citing this article
Maraev V.S. Tools for visualization of time series in space research // The Research of the Science City, 2017, vol. 1, no. 4, pp. 200-207. doi: 10.26732/2225-9449-2017-4-200-207
This Article is licensed under a Creative Commons Attribution-NonCommercial 4.0 International License (CC BY-NC 4.0).