Article
Cover
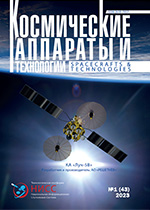
Title
The use of a neural network in solving problems of recognition and classification of spacecraft by their optical imagesAuthors
1K.I. Kushchenko, 2Yu.V. Zheleznyakov, 2A.V. Voloshchuk, 3A.A. Filonov, 3A.A. TolmachevOrganizations
1Military unit 03863Chekhov, Moscow oblast, Russian Federation
2Mozhaisky Military Space Academy
Saint Petersburg, Russian Federation
3Military Aerospace Defense Academy
Tver, Russian Federation
Abstract
In recent years, the number of space objects located in near-Earth outer space, especially in the near operational zone, has increased significantly due to the build-up of space groupings, including dual-use (for example, Starlink) and the remnants of their vital activity (space debris). This factor increases the importance of the task of recognizing and classifying space objects by type in the shortest possible time and entering them into the main catalog of space objects. The developed methodology allows automated analysis of optical images of space objects using software to solve the problem of their recognition and classification by type using a convolutional neural network. The purpose of the study is to increase the efficiency of processing and analysis of optical images of spacecraft. The experimental results of the study confirm the achievement of the research goal. The developed methodology contributes to the development of software and hardware for image processing and can be used in calculations and data preparation for information support of interested officials. For the first time, a training set for a convolutional neural network has been prepared using real optical images of spacecraft obtained in the visible range.Keywords
neural network, spacecraft, optical images, classificationReferences
[1] Kupriyanov N. A., Logunov S. V., Hegai D. K., Sidorov B. P., Shpak A. V. Model' ocenivaniya informativnosti vysokoshirotnogo tra-ektornogo izmeritel'nogo kompleksa [A model for evaluating the informativeness of a highlatitude instrument measuring complex] // High-tech technologies, 2021, no. 3, pp. 89–97. (In Russian)
[2] Caterpillar Ya. N., Mishukov O. A., Orkin V. V., Korobkov A. A. Metodika raspoznavaniya manevriruyushchih kosmicheskih apparatov na osnove nejrosetevoj klassifikacii radiolokacionnyh izobrazhenij [Methods of recognition of maneuvering spacecraft based on neural network classification of radar images] // Proceedings of the Institute of Engineering Physics, 2020, no. 4 (58), pp. 38–39. (In Russian)
[3] Denisov A. V., Popov V. V., Logunov S. V., Karev P. V. Optiko-elektronnyj kompleks detal'nogo nablyudeniya [Optoelectronic complex of de-tailed observation] // Scientific and Technical Bulletin of Information Technologies, Mechanics and Optics, 2020, no. 1, pp. 24–31. (In Russian)
[4] Sozykin A. V. Obzor metodov obucheniya glubokih nejronnyh setej [Review of methods of teaching deep neural networks] // Bulletin of SUSU. Series: Computational Mathematics and Informatics, 2017, no. 3, pp. 28–59. (In Russian)
[5] LeCun Y., Bottou L., Bengio Y., Haffner P. Gradient-based learning applied to document recognition // Proceedings of the IEEE, 1998, no. 11, pp. 2278–2324.
[6] Tsitsulin A. K., Bobrovsky A. I. Gorizonty razlicheniya ob"ektov sistemami videokontrolya sblizheniya kosmicheskih apparatov [Horizons of object discrimination by video monitoring systems of spacecraft approach] // Voprosy Radioelectronics. Series: Television Technology, 2022, issue 3, pp. 3–14. (In Russian)
[7] Ralf Vandebergh. Available at: twitter.com/ralfvandebergh/status/1252942466254323712?lang=gl (accessed 27.02.2022).
[8] Tsitsulin A. K., Bobrovsky A. I., Morozov A. V. Sintez kosmicheskoj videoinformacionnoj sistemy pri shirokom diapazone izmeneniya dal'nosti do ob"ekta [Synthesis of a space video information system with a wide range of range changes to the object] // Voprosy Radioelectronics. Series: Television technique, 2020, issue 2, pp. 19–37. (In Russian)
[9] Oltean M., Muresan H. Fruit recognition from images using deep learning // Acta Univ. Sapientiae, Informatica, 2018, no. 1, pp. 26–42.
[10] Kuchma M. O., Voronin V. V., Bloshchinsky V. D. Nastrojka, obuchenie i testirovanie svertochnoj nejronnoj seti v zadache tematicheskoj obrabotki sputnikovyh izobrazhenij [Setup, training and testing of a convolutional neural network in the task of thematic processing of satellite images] // Informatics and control systems, 2021, no. 1 (67), pp. 94–95. (In Russian)
[11] Caterpillar Ya. N., Zavalishin M. A., Pestun U. A. Modelirovanie informacionnyh sredstv kontrolya kosmicheskogo prostranstva, funkcioniruyushchih v usloviyah dinamicheski izmenyayushchejsya kosmicheskoj obstanovki [Modeling of information means of space control functioning in a dynamically changing space environment] // Proceedings of the Military Space Academy named after A. F. Mozhaisky, 2011, no. 632, pp. 44–49. (In Russian)
For citing this article
Kushchenko K.I., Zheleznyakov Yu.V., Voloshchuk A.V., Filonov A.A., Tolmachev A.A. The use of a neural network in solving problems of recognition and classification of spacecraft by their optical images // Spacecrafts & Technologies, 2023, vol. 7, no. 1, pp. 51-59. doi: 10.26732/j.st.2023.1.06
This Article is licensed under a Creative Commons Attribution-NonCommercial 4.0 International License (CC BY-NC 4.0).